The Walter Mitty Respondent
Why voting intention polls tell different stories.
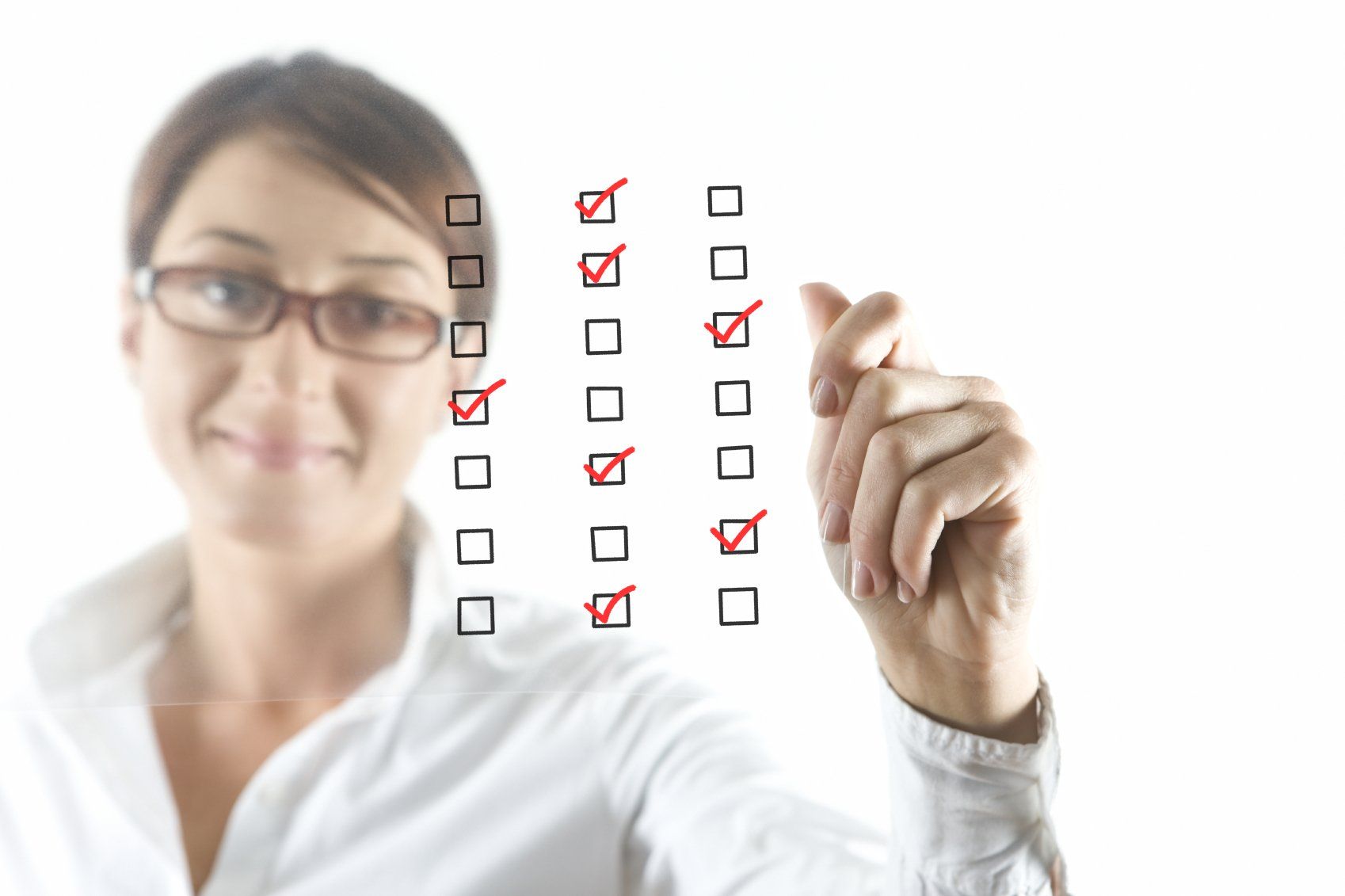
Voting intention polls are a useful tool for assessing public sentiment and predicting election outcomes. But they don't always tell the same story. In the UK general election, opinion polls give Labour a lead of anywhere between 15 points and 23 points. Conservatives are either early polling in the mid 20s or late teens in percentage and Reform either on 13% or 21% depending on which poll you look at (as at 2nd July 2024).
Why is the variability in polling data so great? Several factors contribute to this variability, each rooted in the methodologies and approaches adopted by different polling companies.
This article explores some of these key factors that could be in play to explain why poll results can differ so significantly.
Dealing with "Don't Knows"
One of the primary sources of variability in poll results is how polling companies handle respondents who indicate they "don't know" their voting intention. Different approaches can yield markedly different outcomes:
- Exclusion: Some pollsters exclude "don't know" responses from their final results, reporting only the preferences of decided voters. This method can inflate the percentage shares of the parties.
- Proportional Redistribution: Other companies redistribute "don't know" responses based on the preferences of decided voters, assuming undecided voters will eventually break down similarly.
- Separate Category: Some firms report "don't know" as a distinct category, providing insight into the level of indecision among the electorate but potentially lowering the percentage shares of decided voters.
- Historical Allocation: This method allocates "don't know" responses based on historical voting patterns or demographic correlations, which can introduce assumptions that may not hold in the current context.
Each of these methods can lead to different reported levels of support for parties, contributing to the variability in poll results.
Certainty
Pollsters often assess the certainty of respondents' voting intentions to gauge the firmness of their preferences. Respondents might be asked to score how certain they are to actually vote or might express varying levels of certainty, from "definitely will vote" for a party to "leaning towards" a choice. Polling companies might:
- Weight by Certainty: Give more weight to respondents who express a high certainty in their voting intention. This approach can amplify the support for parties with more committed supporters.
- Report Separately: Some pollsters might report certainty levels separately, providing a nuanced view of voter intention but complicating direct comparisons between polls.
Certainty weighting can significantly affect poll outcomes, especially in close races where the distribution of "leaners" can sway the overall picture.
House Effect
House effect refers to systematic biases that different polling companies may have due to their unique methodologies. These biases can stem from:
- Sampling Techniques: Differences in how respondents are selected can introduce bias. For example, some companies might over-represent certain demographic groups.
- Question Framing: Subtle differences in how questions are phrased or ordered can lead to consistent biases.
- Full or Partial listing: Some agencies will fully list the parties that can be voted for, others will only list the main parties and have 'Other' for those not on the main screen. Clicking on 'Other' then reveals the list of minor parties. Having all parties listed on the first screen can result in higher awareness and voting intention for small parties.
Understanding house effects is crucial for interpreting poll results, as these biases can persist across multiple polls conducted by the same company.
Weighting the Data
Weighting is a critical component of polling methodology, used to ensure the sample accurately reflects the population. Polling companies may weight their data based on:
- Demographics: Adjusting for age, gender, region, and other demographic factors to match population statistics.
- Past Voting Behaviour: Weighting based on respondents' past voting behaviour to predict future actions.
- Different weighting schemes can lead to different results, as slight variations in demographic assumptions or past behaviour models can shift the reported support for parties.
"Walter Mitty" Panellists
Online research panels are increasingly popular, but they can be susceptible to "Walter Mitty" panellists—respondents who misrepresent themselves or provide unreliable data.
The issue of "Walter Mitty" panellists has a large impact on online surveys as samples are predominantly drawn from panels of people who have signed up with the agency. These respondents, named after the fictional character known for living in a fantasy world, can severely distort poll results.
Understanding "Walter Mitty" Panellists
"Walter Mitty" panellists are individuals who misrepresent themselves or provide unreliable responses in online surveys. Their motivations can vary widely, including:
- Incentive Exploitation: Many online panels offer incentives such as cash or points redeemable for rewards. This can lead some respondents to provide false information to qualify for more surveys and maximise their rewards.
- Boredom or Malice: Some people might participate in surveys as a form of entertainment or to intentionally provide misleading answers.
- Attention-Seeking: Just as Walter Mitty escapes into a world of fantasy, some panellists may embellish their responses to appear more interesting or to create a more dramatic narrative.
Consequences for Polling Accuracy
The presence of Walter Mitty panellists can introduce several issues that compromise the reliability and validity of poll results:
- Demographic Misrepresentation: If panellists lie about their age, gender, income or other demographic details, the sample may not accurately reflect the population. This misrepresentation skews the weighting process, leading to inaccurate results.
- Biased Responses: False answers to key questions can distort the findings. For example, a panellist might claim to support a party they have no real intention of voting for, affecting the perceived level of support for that party.
- Inconsistent Data: Repeated participation by Walter Mitty panellists across multiple surveys can lead to data inconsistencies, making it difficult to track genuine trends over time.
- Questionnaire Fatigue: Panellists who rush through surveys to earn rewards quickly may not provide thoughtful or accurate responses, contributing to poor data quality.
While exact quantifications of the "Walter Mitty" problem vary, available data and industry reports suggest it is a significant issue affecting a substantial proportion of online survey responses. Industry reports indicate that up to 20-30% of online panel respondents may engage in behaviours that compromise data quality, including providing inconsistent answers, speeding through surveys or falsifying demographic information. Other research puts this figure much higher. A study in 2020 found that 46% of respondent answers were so poor they couldn't be used.
Similarly, surveys of research professionals have noted that 43% of researchers see "panel quality" as a significant issue, underscoring widespread concern about unreliable respondents.
The Walter Mitty problem is amplified because many people belong to more than one panel and repeat the behaviour across three, four and even five different agency panels. Their presence inflates panel sizes artificially and dilutes the quality of insights derived from genuine respondents. It's a huge problem that is not getting the attention from the industry it requires. Addressing this issue is crucial to maintain the credibility of online research panels.
Conclusion
The variability in voting intention polls highlighted by factors such as handling of "don't know" responses, certainty weighting, house effects, and the influence of unreliable respondents poses significant challenges to the reliability of these polls. This variability raises critical questions about their utility as predictive tools and the extent to which the public and policymakers can confidently rely on them.
In practical terms, the wide range of outcomes from different polls can create confusion rather than clarity, potentially influencing public perception, media narratives, and even strategic decisions by political parties. Moreover, if methodologies are not standardised or transparently communicated, there is a risk that polling results could inadvertently sway voter behavior rather than reflect it accurately.
The presence of "Walter Mitty" panellists in online surveys further complicates matters, casting doubt on the integrity of data gathered. The potential for demographic misrepresentation and biased responses undermines the foundational assumption that polls accurately reflect the broader electorate.
Ultimately, while voting intention polls remain a valuable barometer of public sentiment, their interpretation requires a more transparent reporting of the methodologies employed and the inherent limitations posed by methodological variability and respondent reliability.