How reliable is an online survey?
Getting the balance between speed and accuracy is vital
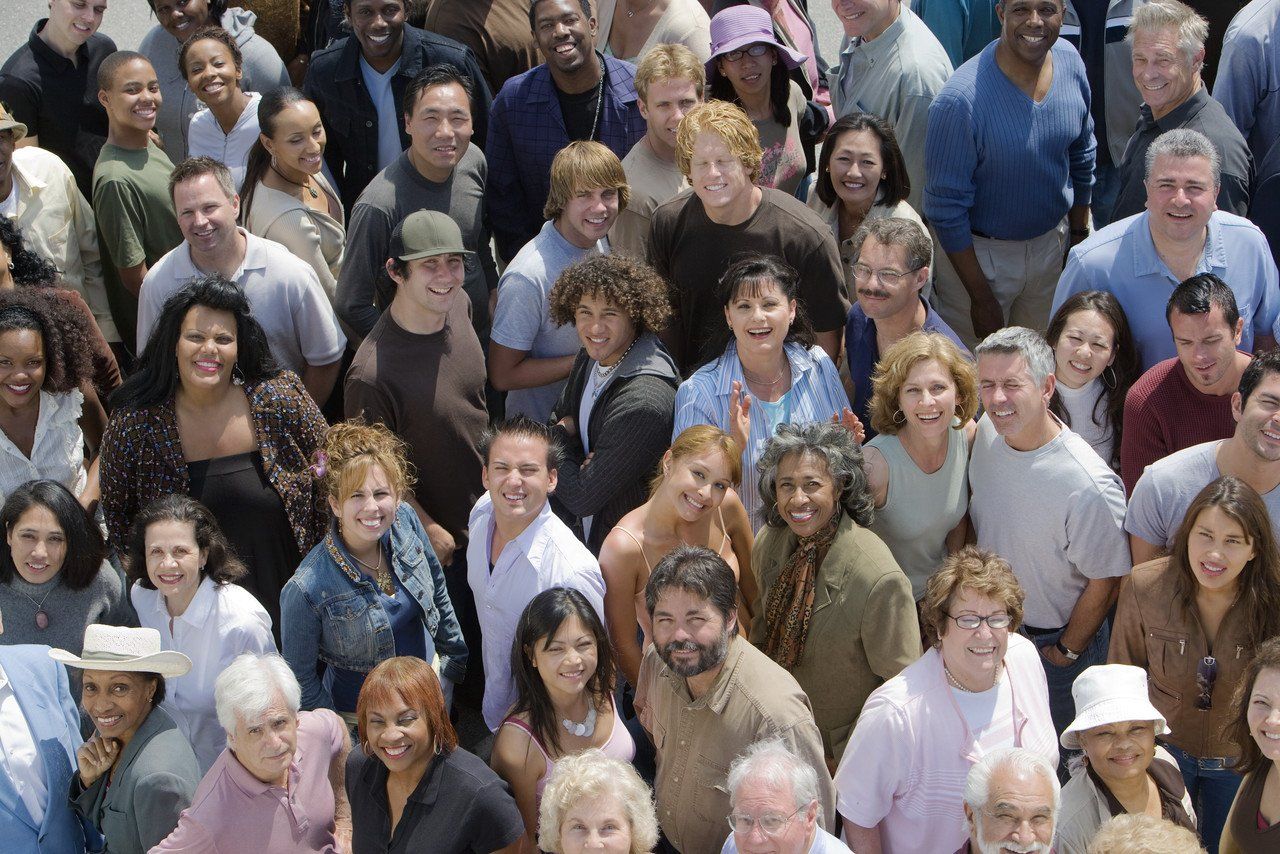
In the age of digitalisation, the allure of online surveys for businesse, especially the DIY platforms, is undeniable. These provide a way to quickly gather data, reach a global audience and do so in a manner that is often more cost-effective than traditional methods like face-to-face or postal surveys.
Beyond ease of use, the real-time data collection allows businesses to respond swiftly to insights. Moreover, the multimedia capabilities of online platforms make them versatile, letting researchers craft surveys that are both engaging and varied.
However, with these advantages come certain concerns.
One of the most pronounced challenges with online surveys is the potential for self-selection bias. Respondents who voluntarily participate might already have a vested interest in the topic, which could skew the results. Furthermore, the so-called 'digital divide' remains a real issue; not everyone has access to the internet, which might inadvertently exclude specific demographic segments from participating. There's also the question of sincerity and authenticity. The veil of online anonymity sometimes encourages non-genuine responses. And of course, in a world flooded with online content, survey fatigue is a genuine concern; respondents can easily feel overwhelmed or indifferent.
Can the accuracy of an online survey be measured?
Statistical measures of survey accuracy are often applied to online surveys. Strictly speaking applying measures such as sampling error to an online survey is mistaken as most online surveys use what is known as a non-probability sample. In a probability survey, every person in the population has a known, non-zero chance of being selected. This allows for direct generalisations from the sample to the population. Standard errors, confidence intervals and significance tests are straightforward to compute.
For non-probability surveys, not all people in the population have a known or consistent chance of being selected. Online surveys often sample through a panel of members who have signed up to do surveys and these might not be representative of the larger population. Because of this, traditional measures of accuracy like margins of error don't have the same straightforward interpretability.
So what can be done to improve the reliability of online surveys?
There are a number of things that can be undertaken pre and post survey that will improve the reliability of the results.
Pre survey activities include:
- Preventing multiple entries from the same individual is vital. Tools like cookies or IP tracking, used within the confines of privacy regulations, can help in maintaining the survey's integrity.
- Before full launch, it's advisable to pre-test the survey on a small group. This can help in ironing out ambiguities and ensure the survey is free from technical hitches.
- To ensure that respondents are genuinely engaged and not just skimming through, attention checks can be embedded in surveys. Such questions require careful reading and can filter out bots or inattentive participants. Along the same lines, ensuring the anonymity and privacy of respondents can boost honest participation. Being transparent about data usage and storage protocols, can foster trust.
- Survey design optimisation is paramount. A concise survey reduces respondent fatigue and clear, simple language aids comprehension. Adaptive questioning, where the survey evolves based on prior responses, can also keep participants intrigued. We will cover question in the next article in this series.
Once the results are in, we suggest doing the some or all of the following:
- Weighting: One common practice is to weight survey results so they align more closely with the known population on key demographic or other variables. However, even with effective weighting, there might still be unknown biases in the results because certain groups might be underrepresented.
- Bootstrap Methods: Resampling techniques like bootstrapping can be used to estimate variability in non-probability samples. By repeatedly drawing samples (with replacement) from the observed data and calculating the desired statistic for each sample, you can get an empirical distribution of the statistic and its variability.
- Model-Based Approaches: Instead of drawing inference from the design of the survey, a model-based approach assumes that a correctly specified model can adjust for the biases in non-probability samples. For example, post-stratification is a technique where responses are adjusted based on known population totals in subgroups.
- Benchmarking: If there are other, more reliable data sources available (e.g., government statistics or probability surveys on similar topics), non-probability survey results can be compared or benchmarked against these. If they align well, it increases confidence in the non-probability results.
One final thing to keep in mind is that when reporting results from online surveys, it's essential to be transparent about the method, including how participants were recruited, potential sources of bias and any steps taken to adjust or weight the data.
In conclusion, while online survey samples present unique challenges, their potential as a data collection tool is vast. By understanding the limitations and proactively enhancing accuracy, they can serve as invaluable assets for businesses and researchers alike